Amazon Web Services Feed
Serverless at re:Invent 2020 – hot takes #1
During this re:Invent, I’m sharing with you the biggest serverless-related announcements and what they mean for you in a series of hot-takes.
Starting with, the biggest announcements from today’s keynote, plus a bunch of other important announcements just before r:Invent.
1. Lambda changes billing to per ms
Lambda now bills you by the ms as opposed to 100 ms. So if your function runs for 42ms you will be billed for 42ms, not 100ms.
This instantly makes everyone’s lambda bills cheaper without anyone having to lift a finger. It’s the best kind of optimization!
However, this might not mean much in practice for a lot of you because your Lambda bill is $5/month, so saving even 50% only buys you a cup of Starbucks coffee a month.
Still, that’s a FREE cup of coffee!
Before, because Lambda bills in 100ms blocks, there’s no point in optimizing if your avg duration is sub 100ms.
NOW, you can micro-optimize and every ms saved is another $0.00000…X saved.
The question is, should you do it?
In 97% of cases, it’d be premature optimization.
As I discussed in this thread, don’t do it until you know your return-on-investment (ROI).
Given all the excitement over Lambda’s per-ms billing change today, some of you might be thinking how much money you can save by shaving 10ms off your function.
Fight that temptation ????until you can prove the ROI on doing the optimization.#serverless #aws #awslambda
— Yan Cui is making the AppSync Masterclass (@theburningmonk) December 1, 2020
Ask yourself these questions:
- how many times is this function invoked a month?
- how much time (in ms) can you save per invocation?
- how much memory does this function need?
From there, you can work out a ballpark figure for how much you stand to save.
Then estimate how many hours is it gonna take you to optimize the code.
Times that by 2 😉
Then look at your wage, how much you’re paid per hour?
Times that by 2, because once bonuses, pensions and everything is factored in, usually an employer ends up paying 2x your salary.
Now, you have both sides of the equation and can work out the ROI for doing this optimization work.
Let me invoke my inner Knuth and to make sure you see the 2nd half of his quote.
If you can identify that critical 3% of use cases (based on ROI, not by how excited you feel about optimizing that bit of code), then you should absolutely do it, especially now you have 10GB functions!
2. Lambda supports 10GB memory and 6 vCPU
Lambda now supports up to 10GB of memory and 6 vCPU cores.
This is another big one for those of you doing HPC or otherwise compute or memory-intensive applications such as training ML models.
Between this, and the AVX2 instruction set (see below), the Lambda team is really starting to look after the Lambda as a supercomputer crowd.
Would love to see more use cases around this, and if anyone fancy talking about what you’re doing then join me on the Real-World Serverless podcast.
3. Aurora Serverless v2
Aurora Serverless v2 was arguably the biggest announcement from today’s opening keynote.
Honestly, it sounds great!
But then again, so did Aurora serverless v1… the devil was in the details. So I’ll reserve my enthusiasm until I see it in action.
And I’m just as curious about what hasn’t been said as what has – there was no mention about cold starts, which was the thing that made everyone go “yuck, we can’t have that in production!”.
And what about data API? I imagine (and hope) that is still available on v2.
4. Lambda supports container images
Lambda also added support for container images as a packaging format.
This is another big announcement and I expect to see lots of excitement and confusion around this.
I’ve shared some details on how it works in this post, which also mentions some use cases and my thoughts on it, please go and check it out.
For better or worse, it’s what people have been asking for.
And for organizations with strict compliance and audit requirements, being able to use the same tooling as their container workload is a must. This is designed for them.
But what’s in it for the rest of us?
Well, it lets you ship a container image up to 10GB, so you can pack a lot of read-only data with your image. Like a giant Machine Learning model, which would have been slow and painful to read from EFS.
BUT, for functions that are shipped as zip files, the deployment package size limit is still 250MB unzipped.
When you use container images, you’re responsible for securing and patching the base image. Even if you use an AWS-provided base image, you still have to deploy their updates to your functions regularly. This is a big set of responsibilities to take on but Ben Kehoe has a great idea on how it can be fixed. It would address my biggest concern with using container images for sure.
This is the feature that containers on Lambda need: the ability for AWS to automatically update the managed base image you’re using (if you’re using one of their images) for your deployed code (if you opt in to it). This is what they can do with zips. https://t.co/2MuB3C8bBG pic.twitter.com/Dgfh5McWDD
— Ben Kehoe (@ben11kehoe) December 1, 2020
However, you’re still much better off by keeping things simple as much as you can and stick to basics.
Using zip files = no building docker images = simpler pipeline (no need to publish to ECR) = no need to worry about function needing optimization again after 14 days of idle (see my post above for more details of the function lifecycle).
I keep saying it, keep it simple for as long as you can, and only reach out for more complex solution when you must.
me: yes!
X: why..?
me: because simplicity wins and most of the time good enough is good enough ???? You should start with the simplest solution and make it more complex when you need to, not the other way around— Yan Cui is making the AppSync Masterclass (@theburningmonk) November 27, 2020
5. AWS Proton
AWS launched the Proton service for deploying container and serverless applications.
Honestly, I’m not sure about this one… there are already lots of ways to deploy serverless and container applications on AWS with both official and 3rd tools.
And looking at the example in this thread, it’s doing deployment by clicking in the AWS console.
Here’s why I think AWS Proton is super rad (a thread): Check out this fun endpoint I deployed today:https://t.co/bNUZiU568G#reInvent #AWS #awsproton
(1/8)— David Killmon ?? (@kohidave) December 1, 2020
Didn’t we learn that was a bad idea 10 years ago?
Maybe I’ve misunderstood the intended target user for this service?
I mean, you have Amplify for frontend developers who don’t wanna know what AWS resources are provisioned.
You have the Serverless framework, AWS SAM, Terraform, AWS CDK and too many others to list here that caters for backend developers who want more say on what’s deployed in your AWS account.
But it’s not clear to me who is Proton designed for.
Surely not your DevOps team or sysadmins, they’re gonna want IaC for sure.
And according to this thread, the UX is not quite ready either (at least not compared to other solutions on the market).
The UX for AWS Proton is “not good” … being kind here.
— Ant Stanley #BLM (@IamStan) December 1, 2020
6. AWS Glue Elastic Views
AWS also announced the preview for AWS Glue Elastic Views.
“Combine and replicate data across multiple data stores using SQL”
This sounds like it has some interesting possibilities! I mean, “materialized views” comes right out of the CQRS book, doesn’t it?
I have built this type of materialized views by hand so many times before. Either in real-time with Kinesis Analytics, or with some sort of batch-job against Athena (where all the raw events are dumped into). This could make all those home-grown solutions redundant.
The fact that it supports S3, ES, RDS, DynamoDB, Aurora and Redshift is pretty insane. I guess one challenge with mashing together all these data sources is that it’s impossible to guarantee ordering of data updates in those systems is respected during replication.
I think the devil is gonna be in the details for this one. But I’m excited about what it could do in any case.
7. S3 replication supports multiple destinations
You can now replicate S3 objects from one source bucket to multiple destination buckets.
This solves a pretty clear (and specific) problem here, that if you need to fan-out the S3 object from one bucket to multiple buckets.
It hasn’t come up a lot in my work, but it’s a blocker when you need that. The way I’ve solved it until now is to use Lambda to do the fan-out, which is a bit wasteful.
8. S3 adds read-after-write consistency for overwrites
S3 now supports read-after-write consistency for operations that overwrites an object.
This is BIG.
Before, read-after-write consistency was only guaranteed for new objects, not for updates. This causes SOOO MANY eventual consistency problems that you end up solving at the app level.
I think Gojko is really gonna appreciate this update. We were talking a while back on my podcast and this was one of the biggest problems he had with S3
9. Amplify launched admin UI
The Amplify team has launched admin UI, a way for you to manage your full-stack application in Amplify with an… well, admin UI.
I had a preview of this last week, and although I don’t use the Amplify CLI, I gotta say this looked pretty slick.
If you use Amplify, you should check it out.
10. S3 supports two-wap replication for object metadata changes
S3 now supports bi-directional replication for object metadata changes such as tags or ACL list.
Notice that this is only for replicating object metadata changes (tags, ACL, etc.) and not the actual data objects themselves.
I’m honestly not sure about the specific use case here…
I get why there isn’t bi-directional data replication, but in what use cases would you be updating metadata in the replicated bucket (the backup/passive bucket, or whatever you’d like to call it)?
That’s it for the big serverless-related announcements from the keynote unless I missed anything.
But there were so many announcements from just before re:Invent.
11. Modules for CloudFormation
AWS announced CloudFormation Modules.
It’s like Terraform modules, but for CloudFormation
I think this one is great, and not just for serverless applications. It gives you a way to create reusable components for a CloudFormation stack, something that AWS tried (and failed, so far) with SAR. Let’s see what happens with modules.
There are some key differences between SAR and modules:
a. modules are private to an account, SARs can be shared publicly.
b. modules are merged into a stack, SARs get compiled into nested stacks.
c. SAR has versioning, modules don’t.
At first glance, it still feels like if your goal is to create reusable components that can be easily shared in your organization, and be able to version control them, then CDK patterns still look the better option here.
Feel free to tell me I’m wrong here.
FWIW, I’m not a fan of CDK, but if I were to use it, it will be because I can create reusable components and distribute them in my org IFF every team is using the same prog language, otherwise, I’d have to replicate the same pattern in every language that teams are using…
12. Lambda Logs API
Lambda released Logs API which works with the Lambda Extensions mechanism that was released in Oct.
This lets you subscribe to Lambda logs in a Lambda extension and ship them elsewhere WITHOUT going through CloudWatch Logs.
Why is it important?
a. It lets you side-step CloudWatch Logs, which often costs more (sometimes 10x more) than Lambda invocations in production applications.
b. It’s possible (although not really feasible right now) to ship logs in real-time
You can disable the Lambda permissions for shipping logs to CloudWatch Logs so they’re never ingested by CloudWatch Logs (and so you don’t pay the data ingestion and storage cost), and use an extension to ship the logs to a log aggregation service (e.g. loggly, logz.io) instead.
There was a bunch of AWS launch partners with official extensions to take advantage of this capability. I expect to see the ecosystem grow around this in the coming months.
Shipping logs in real-time is much trickier. Because a Lambda invocation is not finished until all the extensions are done. So if an extension is busy waiting for logs and shipping them then that adds to the function’s invocation time. Which is bad for any user-facing function such as those behind API Gateway. So it’s not really feasible yet.
What we need is a way to let the function respond while giving the extensions the chance to carry on with some background task, maybe for another couple of ms. Not sure how possible this is, and how that impacts the billing model though.
I have covered Logs API in more detail in this post, which also explains how it works and several other use cases.
13. Code signing
Lambda announced code signing support through AWS Signer. Lambda would check the signature to make sure the code hasn’t been tampered with.
This is probably not something most of you would care about. But in some regulated environments, this might be required to tick a box for audit and compliance requirements. Honestly, I’m glad this is nothing that I’ve ever had to do!
14. AVX2 instruction set
Lambda added support for AVX2 so you can build your app to target the AVX2 instruction set, which lets CPUs do more calculations per CPU cycle.
This is very relevant for those of you who are using Lambda as a cheap, on-demand Supercomputer for scientific computing, Monte Carlo simulations, or any CPU-intensive tasks.
I don’t think it’ll make too much difference to your run-of-the-mill REST APIs. Although maybe you can save a few ms if you need to parse large JSON objects and the extra CPU power can help. And since power == memory == $$ in Lambda, it might save you some Lambda cost too.
That being said, until you’ve benchmarked the difference and proved that it’s worth doing, plz don’t make your life more complicated than it needs to be. It’s really easy to spend $10000 in eng effort to save $10/month with Lambda… Sometimes, good enough is good enough.
15. SQS batch window
Lambda now supports batch window of up to 5 mins for SQS. This lets Lambda batch up SQS messages before invoking your function, so you can now process up to 10,000 SQS messages in one invocation (instead of the default 10 with SQS).
This is great for really high throughput systems, where it’d have otherwise required A LOT more Lambda invocations to process the messages. So you can save on Lambda invocation cost. IF the tasks are not time-sensitive, that is.
There are caveats to consider though.
Messages can be buffered for up to 5 mins before being handed to your function for processing, which might take longer than before because it’s now processing a bigger batch than 10. So you need to adjust the visibility timeout accordingly.
AWS used to recommend using 6x the Lambda timeout for the SQS visibility timeout, I suspect this needs to be updated if you’re using a batch window, maybe it needs to be 6x Lambda timeout + MaximumBatchingWindowInSeconds
.
And you know how partial failures can be tricky?
Well, before you had a batch of up to 10 messages, now you can have a batch of up to 10,000, so partial failures is gonna be even trickier to deal with…
One strategy was to call DeleteMessageBatch
on successful messages yourself and then bubble up the error (see this post for more detail). Now imagine, you have 1 failed message out of a batch of 10,000… so now you need to call DeleteMessageBatch
for 9999 messages, in batches of 10 (SQS limit)…
Would that even work? You’ll probably get throttled, or at the very least, you need a long timeout for the function to cater for “extra time it takes to call DeleteMessageBatch
on 9999 messages”.
In practice, this edge case might never transpire though. I hope…
Maybe in light of this, it’s better to swallow the error and publish the failed messages to a separate queue for the cast-aways where they get retried a few more times (without the batch window) before being sent to a dead-letter queue (DLQ).
16. EventBridge adds SSE and raises default throughput limit
EventBridge now supports server-side encryption (SSE) and upped its default throughput limit to 10,000 events/s in us-east-1
, us-west-2
and eu-west-1
. But I gotta admit, I thought EventBridge had SSE support already! It’s surprising that a service that handles so much user data had gone without SSE support for so many years.
It’s also worth mentioning that EventBridge doesn’t support customer-managed keys (CMKs) yet, which might be a showstopper for regulated environments where the customer has to import their own key materials. Using EventBridge-managed keys also has the same fallacies as SSE-S3 where you don’t need permissions for the KMS key to access the data.
On the other hand, the rate limit raise is definitely good news.
ps. it’s still a soft limit, so you can raise it further if you need to, just pop into the Service Quotas console and raise a request.
17. Sync execution for Step Functions
Step Functions now supports sync workflow executions for Express Workflows. This means you can finally have API Gateway trigger a state machine execution and get the response back without having to poll for it.
This will open up a lot of questions around “should I use a Lambda function or model the biz logic as a state machine in Step Functions?” which deserves a separate blog post to analyze the pros and cons. But I suspect 90% of cases you’d still be better off with Lambda.
But there’s some really cool stuff you can do with Step Functions, like using a callback pattern to wait for a human response or using a Map state to handle map-reduce type workloads, or throw in a good old saga pattern for managing distributed transactions.
18. API Gateway integration for Step Functions
Step Functions adds service integration with API Gateway. This lets you call an API Gateway endpoint (and sign the request with sigv4) without having to use a Lambda function.
This is useful for when you need to call some API Gateway endpoints (most likely internal APIs that uses AWS_IAM auth) as part of a workflow. But as I said before, it’s a shame that it’s limited to API Gateway and not just “any HTTP endpoint”.
It’s great to see Step Functions adding direction integration with API Gateway, but I don’t understand why it’s limited to API Gateway endpoints and not “any HTTP endpoint”? ?
Am I missing something here?https://t.co/WsYcAV6Hgf
— Yan Cui is making the AppSync Masterclass (@theburningmonk) November 17, 2020
19. Better cross-account support for EventBridge
EventBridge makes it easier to manage permissions in a multi-account environment.
This is really significant for many organizations. More and more folks are running a centralised event bus in a separate account. Subscribing and pushing events to this centralised bus was painful and required touching both accounts (for the event bus as well as the event consumer/publisher).
I need to try this out for myself to form an opinion about how well it actually works. But from the docs, it looks like it *should work the way you expect it to.
20. DynamoDB supports PartiQL
DynamoDB adds support for PartiQL.
I’m afraid this sounds like it’s a much bigger deal than it actually is…
First of all, it doesn’t change DynamoDB fundamentally, it hasn’t introduced any new capability beyond “a different syntax to query your data” (albeit a syntax that you might be more comfortable with).
It doesn’t eliminate any of the limitations on how you can query your data.
In fact, there are some dark caveats to look out for.
For instance, it will transparently switch from a Query to a Scan, and we say “friends don’t let friends do DynamoDB scans” for a reason – it’s expensive, inefficient and SLOOOOOWWW.
I get that this feature comes from a good place – to make it easier for folks coming from SQL to adopt DynamoDB. But I feel this is like handing a loaded gun to a baby and pointing it at their feet… it’s gonna be so easy for them to do the wrong thing with this…
And I think it also delays the point when someone realises “I need to learn DynamoDB”, maybe to never! Because they just need to shoot their foot once to run back to RDBMS, and they’re not coming back this time…
21. DynamoDB adds Kinesis Stream
DynamoDB adds support for Kinesis streams, in addition to the existing DynamoDB stream.
I’ve done a separate thread on this already, so maybe check that out first.
Quickly tried out the new Kinesis stream support for DynamoDB, here are some quick observations.https://t.co/EaPehABumm#serverless #aws #dynamodb #nosql
/1
— Yan Cui is making the AppSync Masterclass (@theburningmonk) November 24, 2020
The Good:
- You can use one stream to capture updates from multiple tables.
- You can use Kinesis’s direct integrations with Kinesis Analytics and Firehose.
- You have more control around sharding and throughput.
- You can extend data retention – Kinesis lets you extend retention to up to 1 year.
- You can have more than 2 consumers (DynamoDB streams are limited to 2 consumers), with Kinesis being able to support up to 20 with enhanced fan-out.
The Bad:
- The sequence of records is not guaranteed.
- You can get duplicated events in the stream.
The official doc has a nice side-by-side comparison of the two streaming options.
22. DynamoDB supports export to S3
Liked this article? Support me on Patreon and get direct help from me via a private Slack channel or 1-2-1 mentoring.
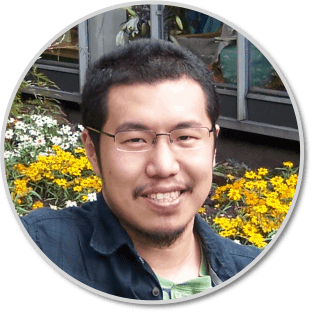
Hi, my name is Yan Cui. I’m an AWS Serverless Hero and the author of Production-Ready Serverless. I specialise in rapidly transitioning teams to serverless and building production-ready services on AWS.
Are you struggling with serverless or need guidance on best practices? Do you want someone to review your architecture and help you avoid costly mistakes down the line? Whatever the case, I’m here to help.
The post Serverless at re:Invent 2020 – hot takes #1 appeared first on theburningmonk.com.